DATA SCIENCE
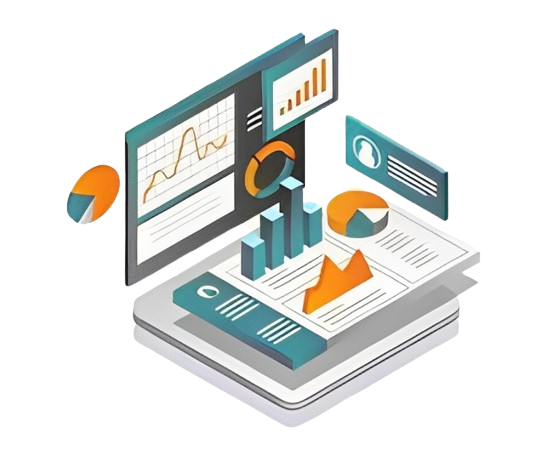
Master the art of transforming data into actionable insights with our Best Data Science Course in Gulbarga.
Duration: 45 Days
- 100% Practical
- Certification
- 7+ Project Assignments
- Agency Style Training
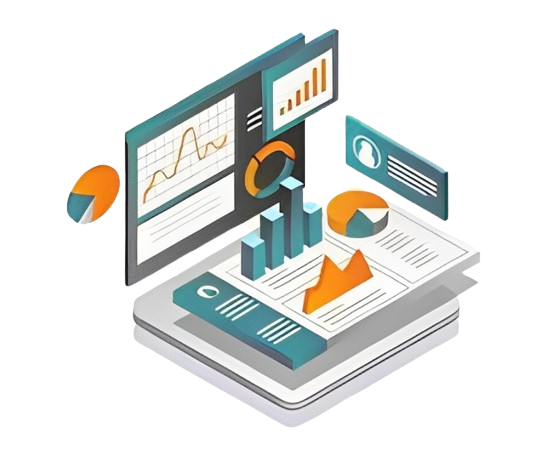
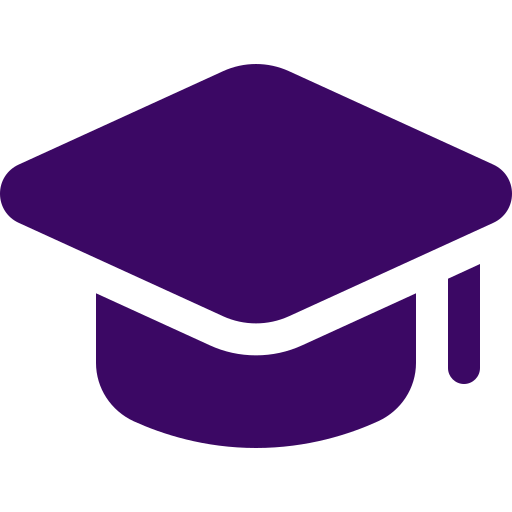
Learn From Agency Specialist
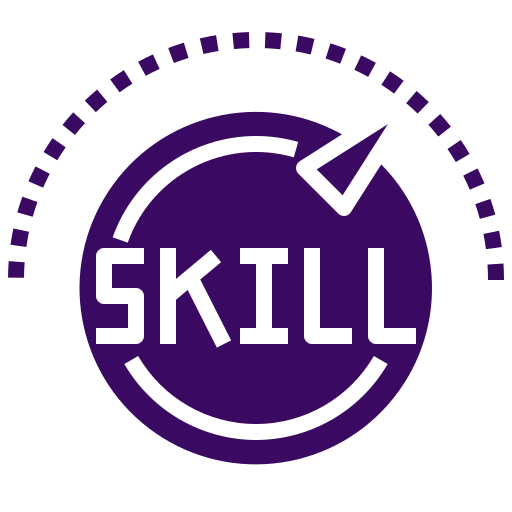
Sharpen skills with learning.
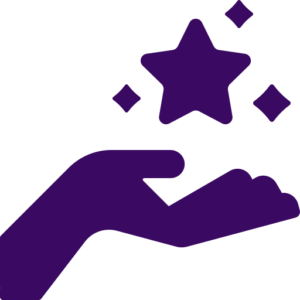
Immersive Hands-On Training
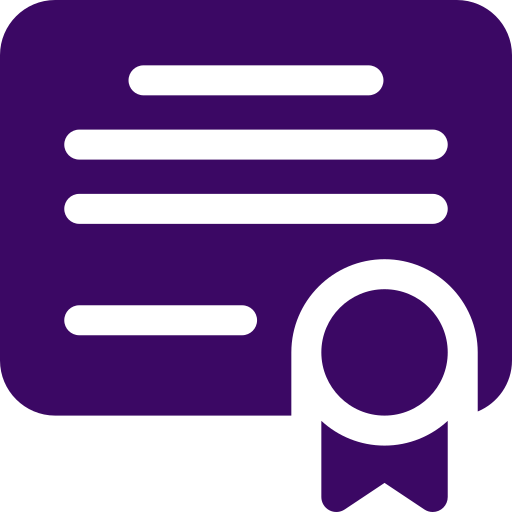
Certification
Data Science Learning Curriculum
Getting Started with Python
- Python Introduction and setting up the environment
- Python Basic Syntax and Data Types
- Use operators like Arithmetic, Logical, and Bitwise for various operations.
- Strings in Python
- Lists
- Tuples
- Sets
- Dictionaries
- For instance, use
if
,if-else
, andif-elif-else
to control program flow based on conditions. - loops like
while
andfor
help execute repetitive tasks. Additionally, usebreak
to exit early orcontinue
to skip iterations. - Getting Started with HackerRank use cases and working on them
- List and Dictionaries comprehension
- Functions
- Anonymous Functions (Lambda)
- Generators
- Modules
- Exceptions and Error Handling
- OOP concepts, such as inheritance, polymorphism, and method overriding, along with features like operator overloading, further enhance Python’s flexibility.
- Manage time-based operations with Python’s date and time functions.
- Use functions like
re.search()
,re.compile()
, andre.find()
for text pattern matching. - Similarly, practice file operations, including opening, closing, reading, and writing, to manage data effectively.
- Learn how APIs connect systems and enable external service integrations.
- Build web applications using Flask, a lightweight web development framework.
- Apply your knowledge with projects like web scraping, sending automated emails, and building a virtual assistant.
Math for DS
- Familiarity with statistics and probability
- Some understanding of linear algebra and calculus
Data Analysis and Visualization Libraries
- Packages (Working on Numpy, Pandas, Matplotlib, Seaborn, etc.)
- Web Scraping (learning about tools, libraries and ethical considerations)
- Exploratory data analysis (EDA)
- Database Access
- SQL
- Power BI
Data Manipulation and Preprocessing
- Data manipulation
- Feature scaling and normalization
- Handling categorical variables
Machine Learning
- Supervised Learning
- Regression (linear regression, polynomial regression, etc.)
- Classification (logistic regression, decision trees, random forests, etc.)
- Unsupervised Learning
- Clustering algorithms (k-means, hierarchical clustering, etc.)
- Dimensionality reduction techniques (PCA, t-SNE)
- Model Evaluation and Validation
- Cross-validation techniques
- Evaluation metrics (accuracy, precision, recall, F1-score, etc.)
Deep Learning
- Artificial Neural networks
- Training neural networks with TensorFlow/Keras (Python)
Times Series
Introduction to Time Series Analysis
Statistical Methods for Time Series Analysis
– Descriptive Statistics: Mean, variance, autocorrelation, partial autocorrelation.
– Decomposition of Time Series: Trend, seasonal, and residual components.
– Smoothing Techniques: Moving averages, exponential smoothing.
Stationarity and Transformation
– Stationarity: Definition, importance, stationarity test (ADF Test, KPSS Test).
– Differencing: Making a time series stationary.
– Log Transformation: Handling non-linear trends.
ARIMA Models
– Autoregressive (AR) Model
– Moving Average (MA) Model
– ARIMA (AutoRegressive Integrated Moving Average) Model
Advanced ARIMA Modeling
– Seasonal ARIMA (SARIMA): Extending ARIMA to seasonal data.
– Model Selection: Criteria (AIC, BIC) and diagnostics.
– Model Validation: Cross-validation techniques.
Other Time Series Models
Exponential Smoothing Methods: Simple Exponential Smoothing, Holt’s Linear Trend Model, Holt-Winters Seasonal Model.
Autoregressive Conditional Heteroskedasticity (ARCH) and Generalized ARCH (GARCH) Models
Machine Learning Approaches to Time Series Forecasting
– Introduction to Machine Learning Models: Overview of machine learning approaches.
– Random Forest and Gradient Boosting: Application to time series data.
– Neural Networks: Recurrent Neural Networks (RNN), Long Short-Term Memory (LSTM) networks.
Time Series Forecasting with Python
– Time Series Forecasting Libraries: Overview of key libraries in Python (e.g., statsmodels, Prophet, pmdarima).
– Hands-on Practice: Implementing various models using Python.
Model Evaluation and Comparison
– Evaluating Forecast Accuracy: Mean Absolute Error (MAE), Mean Squared Error (MSE), Root Mean Squared Error (RMSE), Mean Absolute Percentage Error (MAPE).
– Comparing Models: Techniques for comparing different forecasting models.
Project Work
- Application of learned concepts and techniques to real-world data science projects
- Presentation of project findings and insights